Multi-Focus Image Fusion Using Diffusion Models: A Breakthrough in Image Enhancement
Introduction
Image fusion is a powerful technology that enhances images by solving the depth-of-field problem and capturing all-in-focus images. It has wide-ranging applications and can effectively extend the depth of field of optical lenses. In recent years, deep learning methods for multi-focus image fusion (MFIF) have shown promising results compared to traditional algorithms. Researchers at the Suzhou Institute of Biomedical Engineering and Technology (SIBET) of the Chinese Academy of Sciences (CAS) have taken a fresh approach to image fusion by modeling it as a conditional generation task. They have proposed a novel MFIF algorithm called FusionDiff, based on the diffusion model, which combines the best effect from the field of image generation. This article explores the details of the FusionDiff algorithm and its potential for improving image fusion performance.
The Need for Multi-Focus Image Fusion
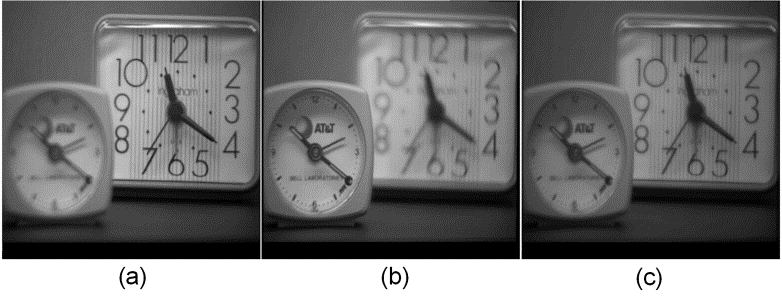
The depth-of-field problem arises when capturing images with different focus points, resulting in some parts of the image being out of focus. MFIF technology addresses this problem by fusing multiple images taken at different focus points to create a single image that is sharp and well-focused throughout. This technology has diverse applications in fields such as medical imaging, surveillance, robotics, and remote sensing.
Traditional MFIF algorithms have limitations in terms of their fusion performance. They often struggle to effectively combine images with varying levels of focus and may introduce artifacts or loss of details in the fused image. Deep learning methods have shown promise in overcoming these limitations by leveraging the power of neural networks to learn complex image representations and fusion strategies.
Introducing FusionDiff: A Diffusion-Based MFIF Algorithm
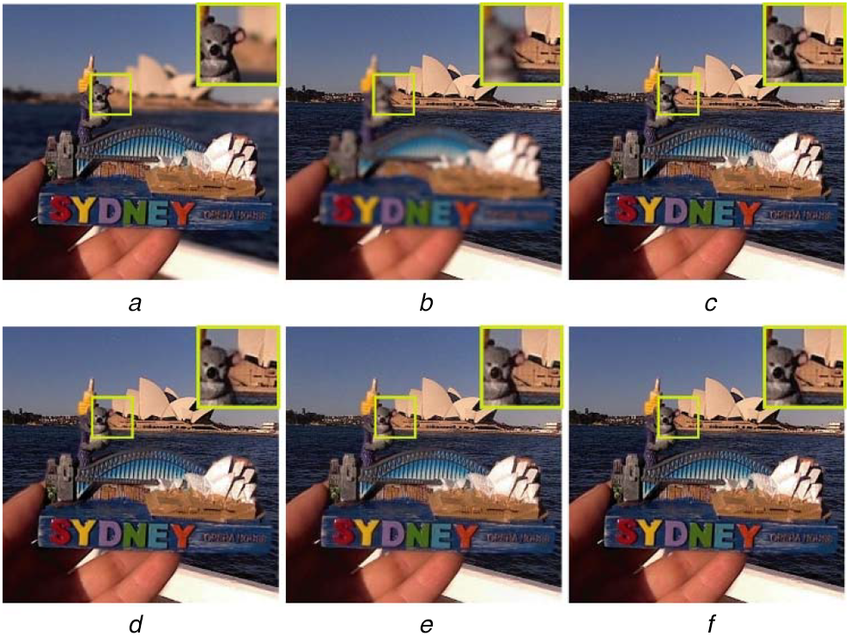
The research team at SIBET has proposed FusionDiff, a novel MFIF algorithm that combines the diffusion model with the best effect from the field of image generation. This approach offers a new perspective on image fusion and opens up new possibilities for improving fusion performance.
Diffusion Model in MFIF
The diffusion model used in FusionDiff is a conditional generation model that learns to transform a set of input images into a fused image. This model uses probabilistic diffusion processes to iteratively update the image pixels based on their local characteristics and the information from neighboring pixels. By applying the diffusion process multiple times, the model progressively improves the fusion quality.
Advantages of FusionDiff
FusionDiff offers several advantages over traditional MFIF algorithms:
- Improved Fusion Performance: FusionDiff outperforms traditional algorithms in terms of image fusion effect and few-shot learning performance. It produces fused images with enhanced sharpness, detail preservation, and overall visual quality.
- Few-shot Learning: FusionDiff is a few-shot learning model, meaning it does not require a large amount of training data to generate high-quality results. This reduces the dependence of the fusion model on the dataset and enables efficient fusion even with limited training samples.
- Model-Driven Fusion: FusionDiff represents a shift from data-driven to model-driven fusion. It leverages the diffusion model to guide the fusion process, making it less reliant on specific training data and more adaptable to various fusion scenarios.
Experimental Results
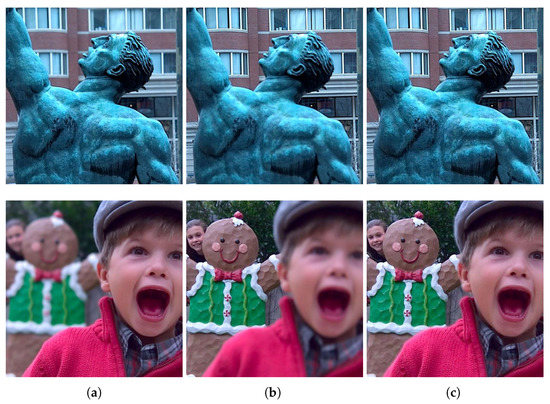
The researchers conducted extensive experiments to evaluate the performance of FusionDiff compared to other MFIF algorithms. The results demonstrated the superiority of FusionDiff in terms of fusion quality and dataset efficiency.
Image Fusion Effect
FusionDiff achieved remarkable image fusion effects, surpassing traditional algorithms. The fused images exhibited improved sharpness, enhanced details, and better overall visual quality. The diffusion model utilized in FusionDiff effectively combined information from multiple input images, resulting in superior fusion performance.
Few-shot Learning Performance
FusionDiff’s few-shot learning capability was a significant advantage. The algorithm achieved the same fusion quality as other algorithms while using only 2% of the training data they employed. This reduced reliance on a large dataset makes FusionDiff more practical and efficient for real-world applications.
Potential Applications and Future Directions
FusionDiff’s improved fusion performance and few-shot learning capability make it a promising tool for various applications. Some potential applications include medical imaging, surveillance systems, robotics, and remote sensing. The researchers believe that FusionDiff’s model-driven approach can be further enhanced and applied to other image enhancement tasks beyond MFIF.
Conclusion
The FusionDiff algorithm represents a significant advancement in multi-focus image fusion. By combining the diffusion model with the best effect from image generation, FusionDiff achieves superior fusion performance and few-shot learning capability. It offers a new perspective on image fusion and reduces the dependence on large training datasets. FusionDiff opens up new possibilities for enhancing image quality in various applications and paves the way for future research in model-driven image fusion algorithms.